Advanced Reproducibility in Cancer Informatics
About this Course
This course introduces tools that help enhance reproducibility and replicability in the context of cancer informatics. It uses hands-on exercises to demonstrate in practical terms how to get acquainted with these tools but is by no means meant to be a comprehensive dive into these tools. The course introduces tools and their concepts such as git and GitHub, code review, Docker, and GitHub actions. Target Audience The course is intended for students in the biomedical sciences and researchers who use informatics tools in their research. It is the follow up course to the Introduction to Reproducibility in Cancer Informatics course. Learners who take this course should: - Have some familiarity with R or Python - Have take the Introductory Reproducibility in Cancer Informatics course - Have some familiarity with GitHub Motivation Data analyses are generally not reproducible without direct contact with the original researchers and a substantial amount of time and effort (BeaulieuJones, 2017). Reproducibility in cancer informatics (as with other fields) is still not monitored or incentivized despite that it is fundamental to the scientific method. Despite the lack of incentive, many researchers strive for reproducibility in their own work but often lack the skills or training to do so effectively. Equipping researchers with the skills to create reproducible data analyses increases the efficiency of everyone involved. Reproducible analyses are more likely to be understood, applied, and replicated by others. This helps expedite the scientific process by helping researchers avoid false positive dead ends. Open source clarity in reproducible methods also saves researchers\' time so they don\'t have to reinvent the proverbial wheel for methods that everyone in the field is already performing. Curriculum The course includes hands-on exercises for how to apply reproducible code concepts to their code. Individuals who take this course are encouraged to complete these activities as they follow along with the course material to help increase the reproducibility of their analyses. **Goal of this course:** To equip learners with a deeper knowledge of the capabilities of reproducibility tools and how they can apply to their existing analyses scripts and projects. **What is NOT the goal of this course:** To be a comprehensive dive into each of the tools discussed. . How to use the course Each chapter has associated exercises that you are encourage to complete in order to get the full benefit of the course This course is designed with busy professional learners in mind -- who may have to pick up and put down the course when their schedule allows. In general, you are able to skip to chapters you find a most useful to (One incidence where a prior chapter is required is noted). Each chapter has associated exercises that you are encourage to complete in order to get the full benefit of the courseCreated by: Johns Hopkins University
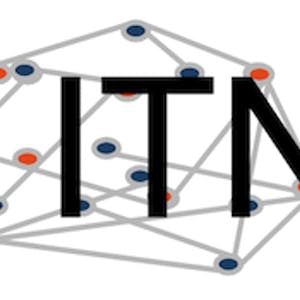
Related Online Courses
The primary objectives of this course are to introduce software engineering techniques and their application to real-world business problems. You will be equipped with practical design and... more
Kursus ini membekali peserta dengan keterampilan untuk membangun solusi yang sangat andal dan efisien di Google Cloud menggunakan pola desain yang telah terbukti. Kursus ini merupakan kelanjutan... more
About GANs\\n\\nGenerative Adversarial Networks (GANs) are powerful machine learning models capable of generating realistic image, video, and voice outputs.\\n\\nRooted in game theory, GANs have... more
This is a self-paced lab that takes place in the Google Cloud console. In this lab you learn how to use Gemini code generation, explanation and suggestions in BigQuery.Created by: Google Cloud more
Bersiaplah untuk karier baru di bidang analitik data yang sedang tumbuh pesat, tidak diperlukan pengalaman atau gelar apa pun di sini. Dapatkan pelatihan profesional yang oleh Google dan manfaatkan... more