Measuring Total Data Quality
About this Course
By the end of this second course in the Total Data Quality Specialization, learners will be able to: 1. Learn various metrics for evaluating Total Data Quality (TDQ) at each stage of the TDQ framework. 2. Create a quality concept map that tracks relevant aspects of TDQ from a particular application or data source. 3. Think through relative trade-offs between quality aspects, relative costs and practical constraints imposed by a particular project or study. 4. Identify relevant software and related tools for computing the various metrics. 5. Understand metrics that can be computed for both designed and found/organic data. 6. Apply the metrics to real data and interpret their resulting values from a TDQ perspective. This specialization as a whole aims to explore the Total Data Quality framework in depth and provide learners with more information about the detailed evaluation of total data quality that needs to happen prior to data analysis. The goal is for learners to incorporate evaluations of data quality into their process as a critical component for all projects. We sincerely hope to disseminate knowledge about total data quality to all learners, such as data scientists and quantitative analysts, who have not had sufficient training in the initial steps of the data science process that focus on data collection and evaluation of data quality. We feel that extensive knowledge of data science techniques and statistical analysis procedures will not help a quantitative research study if the data collected/gathered are not of sufficiently high quality. This specialization will focus on the essential first steps in any type of scientific investigation using data: either generating or gathering data, understanding where the data come from, evaluating the quality of the data, and taking steps to maximize the quality of the data prior to performing any kind of statistical analysis or applying data science techniques to answer research questions. Given this focus, there will be little material on the analysis of data, which is covered in myriad existing Coursera specializations. The primary focus of this specialization will be on understanding and maximizing data quality prior to analysis.Created by: University of Michigan
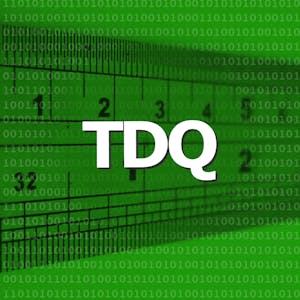
Related Online Courses
This specialization covers the foundations of visualization in the context of the data science workflow. Through the application of interactive visual analytics, students will learn how to extract... more
This is a self-paced lab that takes place in the Google Cloud console. In this hands-on lab you will build, change, provision, and destroy infrastructure using Terraform in the cloud... more
In this course, you will learn about software defined networking and how it is changing the way communications networks are managed, maintained, and secured.Created by: The University of Chicago more
The data science revolution has produced reams of new data from a wide variety of new sources. These new datasets are being used to answer new questions in way never before conceived. Visualization... more
This course on integrating sensors with your Raspberry Pi is course 3 of a Coursera Specialization and can be taken separately or as part of the specialization. Although some material and... more