Interpretable Machine Learning
About this Course
As Artificial Intelligence (AI) becomes integrated into high-risk domains like healthcare, finance, and criminal justice, it is critical that those responsible for building these systems think outside the black box and develop systems that are not only accurate, but also transparent and trustworthy. This course is a comprehensive, hands-on guide to Interpretable Machine Learning, empowering you to develop AI solutions that are aligned with responsible AI principles. You will also gain an understanding of the emerging field of Mechanistic Interpretability and its use in understanding large language models. Through discussions, case studies, programming labs, and real-world examples, you will gain the following skills: 1. Describe interpretable machine learning and differentiate between interpretability and explainability. 2. Explain and implement regression models in Python. 3. Demonstrate knowledge of generalized models in Python. 4. Explain and implement decision trees in Python. 5. Demonstrate knowledge of decision rules in Python. 6. Define and explain neural network interpretable model approaches, including prototype-based networks, monotonic networks, and Kolmogorov-Arnold networks. 7. Explain foundational Mechanistic Interpretability concepts, including features and circuits 8. Describe the Superposition Hypothesis 9. Define Representation Learning and be able to analyze current research on scaling Representation Learning to LLMs. This course is ideal for data scientists or machine learning engineers who have a firm grasp of machine learning but have had little exposure to interpretability concepts. By mastering Interpretable Machine Learning approaches, you\'ll be equipped to create AI solutions that are not only powerful but also ethical and trustworthy, solving critical challenges in domains like healthcare, finance, and criminal justice. To succeed in this course, you should have an intermediate understanding of machine learning concepts like supervised learning and neural networks.Created by: Duke University
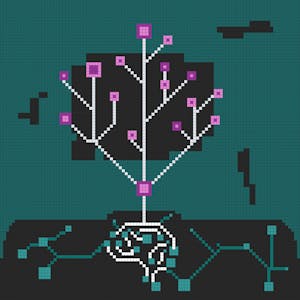
Related Online Courses
This is a self-paced lab that takes place in the Google Cloud console. In this lab, you will learn how to build and deploy an agent (model, tools, and reasoning) using the Vertex AI SDK for... more
In this course, you will learn about the famous dichotomy between active and passive investing, how to appropriately measure and analyze investment performance and what the future trends in the... more
This series of four courses models learning-by-doing through engaging, creative, and collaborative activities as well as conceptual videos that move beyond instructor lectures. The activities and... more
Would you like to spend some time of your study experience or even your whole study course abroad? Did you hear about the top universities in Germany? Then \"Welcome to Munich\"! In the first... more
Join Us in a Top 50 MOOC of All Time!\\n\\nHow do we sequence and compare genomes? How do we identify the genetic basis for disease? How do we construct an evolutionary Tree of Life for all species... more