Decision Making and Reinforcement Learning
About this Course
This course is an introduction to sequential decision making and reinforcement learning. We start with a discussion of utility theory to learn how preferences can be represented and modeled for decision making. We first model simple decision problems as multi-armed bandit problems in and discuss several approaches to evaluate feedback. We will then model decision problems as finite Markov decision processes (MDPs), and discuss their solutions via dynamic programming algorithms. We touch on the notion of partial observability in real problems, modeled by POMDPs and then solved by online planning methods. Finally, we introduce the reinforcement learning problem and discuss two paradigms: Monte Carlo methods and temporal difference learning. We conclude the course by noting how the two paradigms lie on a spectrum of n-step temporal difference methods. An emphasis on algorithms and examples will be a key part of this course.Created by: Columbia University
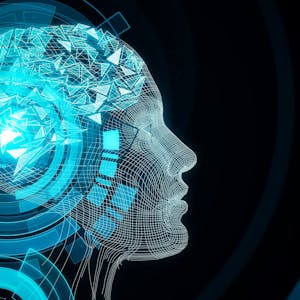
Related Online Courses
In this project we are going to learn about lambda expressions and it\'s application in python. We are going to start with what is Lambda expression and how we can define it, comparing lambda... more
This Specialization is designed for professionals and students seeking a comprehensive understanding of IoT Security. It includes three courses: Foundations of Secure IoT Architecture, Managing IoT... more
Master Agile and Scrum methodologies to drive successful IT project management. This course equips you with the best practices to enhance efficiency, collaboration, and adaptability in dynamic... more
This course is best suited for individuals currently in the healthcare sector, as a provider, payer, or administrator. Individuals pursuing a career change to the healthcare sector may also be... more
This specialization covers common topics in intermediate grammar, such as perfect verb tenses and adjective clauses. It will also cover \"tricky English\" grammar topics that learners of English... more