Decision Making and Reinforcement Learning
About this Course
This course is an introduction to sequential decision making and reinforcement learning. We start with a discussion of utility theory to learn how preferences can be represented and modeled for decision making. We first model simple decision problems as multi-armed bandit problems in and discuss several approaches to evaluate feedback. We will then model decision problems as finite Markov decision processes (MDPs), and discuss their solutions via dynamic programming algorithms. We touch on the notion of partial observability in real problems, modeled by POMDPs and then solved by online planning methods. Finally, we introduce the reinforcement learning problem and discuss two paradigms: Monte Carlo methods and temporal difference learning. We conclude the course by noting how the two paradigms lie on a spectrum of n-step temporal difference methods. An emphasis on algorithms and examples will be a key part of this course.Created by: Columbia University
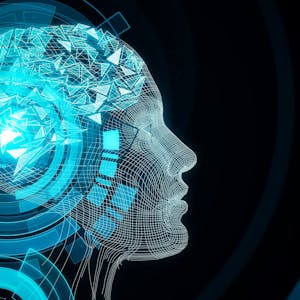
Related Online Courses
This course takes you on a comprehensive journey from the basics of 3D modeling in Blender to importing a game-ready medieval windmill into Unreal Engine 5. Starting with a solid foundation in... more
This is a self-paced lab that takes place in the Google Cloud console. This hands-on lab shows you how to create a small App Engine application that displays a short message. Watch the short video more
This is a self-paced lab that takes place in the Google Cloud console..In this lab, you will learn how to use Datadog Monitoring with Google Eventarc.Created by: Google Cloud more
This course introduces students to the basic components of electronics: diodes, transistors, and op amps. It covers the basic operation and some common applications.Created by: Georgia Institute of... more
This course focuses on integrating sustainability into core business strategies, measuring and reporting sustainability performance, and developing human capital for sustainability. Learners will... more