The Total Data Quality Framework
About this Course
By the end of this first course in the Total Data Quality specialization, learners will be able to: 1. Identify the essential differences between designed and gathered data and summarize the key dimensions of the Total Data Quality (TDQ) Framework; 2. Define the three measurement dimensions of the Total Data Quality framework, and describe potential threats to data quality along each of these dimensions for both gathered and designed data; 3. Define the three representation dimensions of the Total Data Quality framework, and describe potential threats to data quality along each of these dimensions for both gathered and designed data; and 4. Describe why data analysis defines an important dimension of the Total Data Quality framework, and summarize potential threats to the overall quality of an analysis plan for designed and/or gathered data. This specialization as a whole aims to explore the Total Data Quality framework in depth and provide learners with more information about the detailed evaluation of total data quality that needs to happen prior to data analysis. The goal is for learners to incorporate evaluations of data quality into their process as a critical component for all projects. We sincerely hope to disseminate knowledge about total data quality to all learners, such as data scientists and quantitative analysts, who have not had sufficient training in the initial steps of the data science process that focus on data collection and evaluation of data quality. We feel that extensive knowledge of data science techniques and statistical analysis procedures will not help a quantitative research study if the data collected/gathered are not of sufficiently high quality. This specialization will focus on the essential first steps in any type of scientific investigation using data: either generating or gathering data, understanding where the data come from, evaluating the quality of the data, and taking steps to maximize the quality of the data prior to performing any kind of statistical analysis or applying data science techniques to answer research questions. Given this focus, there will be little material on the analysis of data, which is covered in myriad existing Coursera specializations. The primary focus of this specialization will be on understanding and maximizing data quality prior to analysis.Created by: University of Michigan
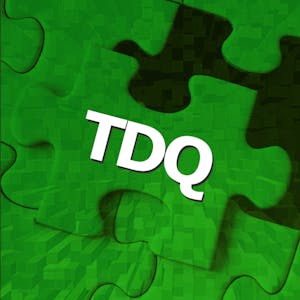
Related Online Courses
In this project, we will analyze life expectancy data by performing data wrangling & exploratory data analysis (EDA). Pandas is a powerful open source data analysis tools in python. Exploratory... more
Test Driven Development (TDD) is an industry accepted technique to build Enterprise Applications and enhance quality. This course will introduce a test first approach for the development of Java... more
In this guided project, you\'ll uncover the secrets to writing efficient and clean Kotlin code by mastering its streamlined syntax features. Perfect for developers with a basic understanding of... more
As machine learning models begin making important decisions based on massive datasets, we need to be aware of their limitations. In this specialization, we will explore the rise of algorithms,... more
Demonstrate your mastery of Java development by completing this capstone project! In this course, you will apply your knowledge and skills to a real-life inspired challenge and use your expertise... more